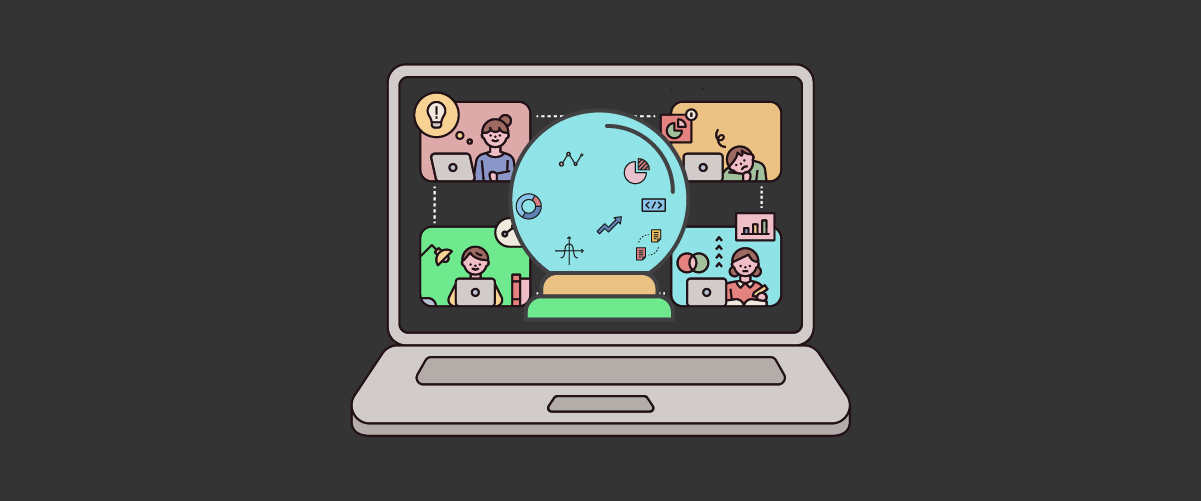
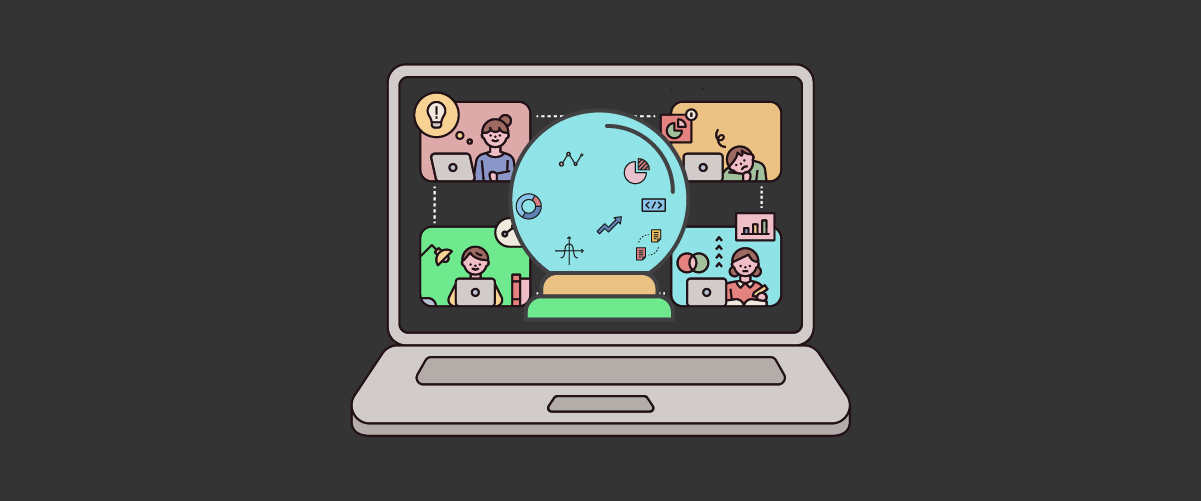
Predictive Analytics in eLearning
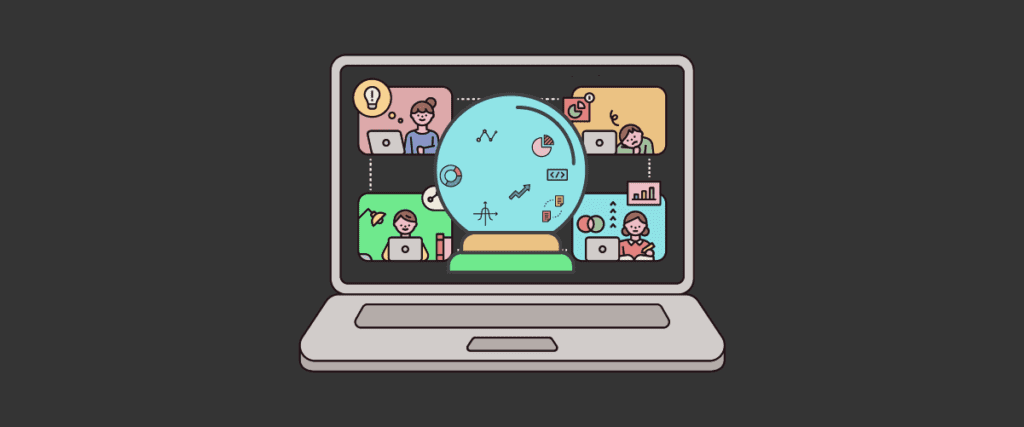
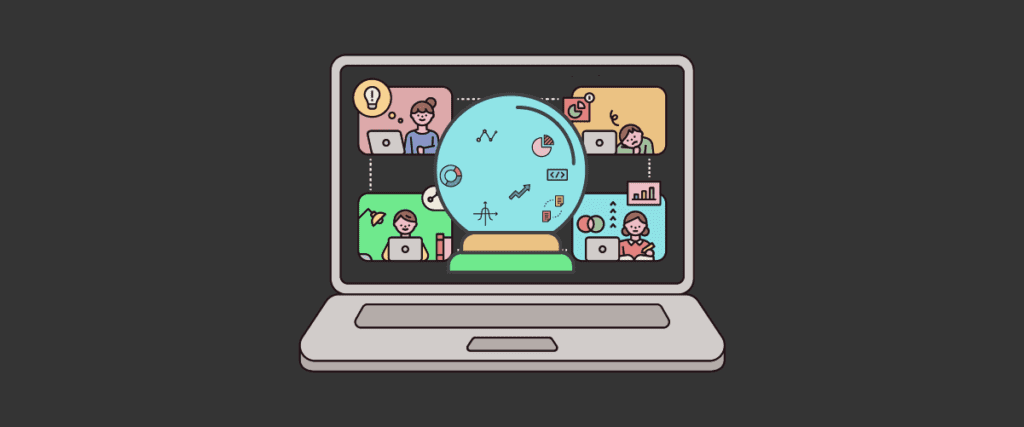
In this short article we will explore the transformative power of predictive analytics in e-learning, delving into its workings, the valuable insights it provides to educators, what kind of data to use in predictive analytics and the tools that facilitate its implementation.
What is predictive analytics in eLearning ?
Predictive analytics in eLearning is the application of data mining and statistical techniques to identify patterns and trends in learner data that can be used to predict future behavior and outcomes. This powerful approach allows educators to proactively address potential challenges and opportunities for improvement, enhancing the overall effectiveness of eLearning programs.
How does predictive analytics in eLearning work?
Predictive analytics in eLearning utilizes historical learner data, such as assessment scores, engagement metrics, time spent on modules, and participation in discussions, to identify patterns and correlations that can inform predictions about future performance. By analyzing these patterns, educators can gain insights into:
- Student Success and Risk: Predictive models can identify students who are at risk of dropping out, struggling with specific concepts, or performing below their potential. This early recognition allows educators to provide targeted interventions, such as personalized support, additional resources, or alternative instructional strategies.
- Course Design and Delivery: Predictive analytics can reveal areas of course content or instructional methods that may be causing confusion, leading to low engagement or poor performance. By identifying these pain points, educators can refine course design, adjust pacing, and improve the overall delivery of instruction.
- Individual Learning Needs and Preferences: Predictive models can help uncover individual learner patterns, such as preferred learning styles, optimal study habits, and areas of strength or weakness. This personalized understanding enables educators to tailor instruction, provide differentiated assignments, and create a more effective learning experience for each student.
- Resource Allocation and Program Effectiveness: Predictive analytics can inform decisions about resource allocation, such as staffing, technology investments, and course development. By understanding how students interact with resources and how these interactions impact outcomes, educators can optimize resource usage and enhance program effectiveness.
What kind of data can be used in Predictive Analytics in e-Learning ?
This data used in predictive analytics in e-Learning can be categorized into three main types:
Learner Demographic Data: This includes information such as age, gender, location, educational background, and prior knowledge or skills. Understanding learner demographics helps educators tailor instruction to different learning styles and experiences.
Learner Activity Data: This encompasses data generated through learners’ interactions with e-learning platforms and content. It includes metrics such as time spent on modules, completion rates, assessment scores, participation in discussions, and use of resources. Analyzing learner activity data reveals patterns in engagement, strengths, and areas for improvement.
Learner Behavioral Data: This involves data that reflects learners’ emotional states, motivations, and attitudes towards learning. It can include data from surveys, feedback forms, online interactions, and facial recognition technology. Understanding learner behavior helps educators identify potential challenges, address emotional barriers, and foster a more supportive learning environment.
What are the tools for predictive analytics?
There are a variety of tools that can be used for predictive analytics in eLearning. Some of the most popular options include:
- Google Analytics: Google Analytics is a powerful web analytics tool that can be used to track a variety of data, including user behavior, website traffic, and conversions. It can be used to identify patterns and trends in data that can inform predictive models.
- IBM SPSS Modeler: IBM SPSS Modeler is a comprehensive statistical analysis and data mining tool that can be used to build and test predictive models. It offers a variety of features that make it well-suited for predictive analytics in eLearning, including data cleaning, feature engineering, and model selection.
- RapidMiner: RapidMiner is an open-source data science platform that can be used for a variety of tasks, including data mining, machine learning, and predictive analytics. It offers a variety of features that make it well-suited for predictive analytics in eLearning, including data preparation, model building, and model evaluation.
- KNIME: KNIME is another open-source data science platform that can be used for a variety of tasks, including data mining, machine learning, and predictive analytics. It offers a variety of features that make it well-suited for predictive analytics in eLearning, including data visualization, model building, and model deployment.
- Microsoft Azure Machine Learning Studio: Microsoft Azure Machine Learning Studio is a cloud-based machine learning platform that can be used to build and deploy predictive models. It offers a variety of features that make it well-suited for predictive analytics in eLearning, including data preparation, model training, and model evaluation.
- Kaggle: Kaggle is a public data science platform that can be used to find and share data, build and train models, and participate in data science competitions. It is a great resource for finding and using predictive models for eLearning.
The best tool for predictive analytics in eLearning will depend on the specific needs and requirements of the organization. Some factors to consider when choosing a tool include:
Cost: Predictive analytics tools can range in price from free to very expensive.
Ease of use: Some tools are more user-friendly than others.
Features: Different tools offer different features.
Support: Some tools offer more support than others.
It is important to consider all of these factors when choosing a predictive analytics tool for eLearning.
Predictive analytics is transforming the eLearning landscape, providing educators with invaluable insights into student behavior, performance, and engagement. As data collection and analysis tools continue to evolve, the potential of predictive analytics in eLearning will only grow, empowering educators to create personalized learning experiences that maximize student success. With its ability to predict future outcomes, predictive analytics is poised to revolutionize the way we teach and learn in the digital age.